Big Data Basics - Part 2 - Overview of Big Data Architecture
Problem
I read the tip on Introduction to Big Data and would like to know more about how Big Data architecture looks in an enterprise, what are the scenarios in which Big Data technologies are useful, and any other relevant information.Solution
In this tip, let us take a look at the architecture of a modern data processing and management system involving a Big Data ecosystem, a few use cases of Big Data, and also some of the common reasons for the increasing adoption of Big Data technologies.Architecture
Before we look into the architecture of Big Data, let us take a look at a high level architecture of a traditional data processing management system. It looks as shown below.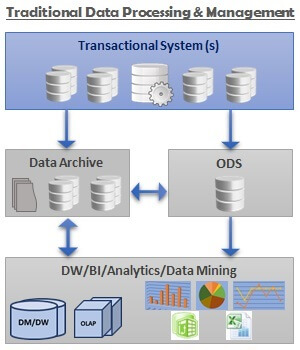
As we can see in the above architecture, mostly structured data is involved and is used for Reporting and Analytics purposes. Although there are one or more unstructured sources involved, often those contribute to a very small portion of the overall data and hence are not represented in the above diagram for simplicity. However, in the case of Big Data architecture, there are various sources involved, each of which is comes in at different intervals, in different formats, and in different volumes. Below is a high level architecture of an enterprise data management system with a Big Data engine.
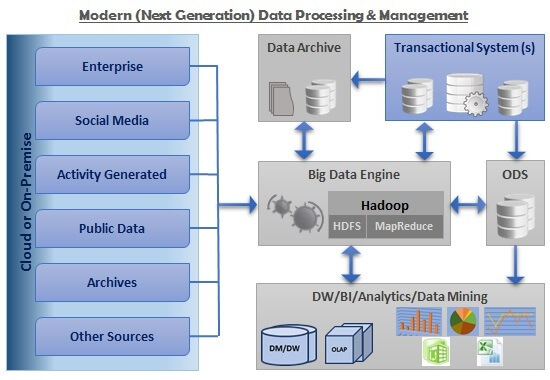
Source Systems
As discussed in the previous tip, there are various different sources of Big Data including Enterprise Data, Social Media Data, Activity Generated Data, Public Data, Data Archives, Archived Files, and other Structured or Unstructured sources.Transactional Systems
In an enterprise, there are usually one or more Transactional/OLTP systems which act as the backend databases for the enterprise's mission critical applications. These constitute the transactional systems represented above.Data Archive
Data Archive is collection of data which includes the data archived from the transactional systems in compliance with an organization's data retention and data governance policies, and aggregated data (which is less likely to be needed in the near future) from a Big Data engine etc.ODS
Operational Data Store is a consolidated set of data from various transactional systems. This acts as a staging data hub and can be used by a Big Data Engine as well as for feeding the data into Data Warehouse, Business Intelligence, and Analytical systems.Big Data Engine
This is the heart of modern (Next-Generation / Big Data) data processing and management system architecture. This engine capable of processing large volumes of data ranging from a few Megabytes to hundreds of Terabytes or even Petabytes of data of different varieties, structured or unstructured, coming in at different speeds and/or intervals. This engine consists primarily of a Hadoop framework, which allows distributed processing of large heterogeneous data sets across clusters of computers. This framework consists of two main components, namely HDFS and MapReduce. We will take a closer look at this framework and its components in the next and subsequent tips.Big Data Use Cases
Big Data technologies can solve the business problems in a wide range of industries. Below are a few use cases.- Banking and Financial Services
- Fraud Detection to detect the possible fraud or suspicious transactions in Accounts, Credit Cards, Debit Cards, and Insurance etc.
- Retail
- Targeting customers with different discounts, coupons, and promotions etc. based on demographic data like gender, age group, location, occupation, dietary habits, buying patterns, and other information which can be useful to differentiate/categorize the customers.
- Marketing
- Specifically outbound marketing can make use of customer demographic information like gender, age group, location, occupation, and dietary habits, customer interests/preferences usually expressed in the form of comments/feedback and on social media networks.
- Customer's communication preferences can be identified from various sources like polls, reviews, comments/feedback, and social media etc. and can be used to target customers via different channels like SMS, Email, Online Stores, Mobile Applications, and Retail Stores etc.
- Sentiment Analysis
- Organizations use the data from social media sites like Facebook, Twitter etc. to understand what customers are saying about the company, its products, and services. This type of analysis is also performed to understand which companies, brands, services, or technologies people are talking about.
- Customer Service
- IT Services and BPO companies analyze the call records/logs to gain insights into customer complaints and feedback, call center executive response/ability to resolve the ticket, and to improve the overall quality of service.
- Call center data from telecommunications industries can be used to analyze the call records/logs and optimize the price, and calling, messaging, and data plans etc.
Big Data Adoption
Data has always been there and is growing at a rapid pace. One question being asked quite often is "Why are organizations taking interest in the silos of data, which otherwise was not utilized effectively in the past, and embracing Big Data technologies today?". The reason for adoption of Big Data technologies is due to various factors including the following:- Cost Factors
- Availability of Commodity Hardware
- Availability of Open Source Operating Systems
- Availability of Cheaper Storage
- Availability of Open Source Tools/Software
- Business Factors
- There is lot of data being generated outside the enterprise and organizations are compelled to consume that data to stay ahead of the competition. Often organizations are interested in a subset of this large volume of data.
- The volume of structured and unstructured data being generated in the enterprise is very large and cannot be effectively handled using the traditional data management and processing tools.
References
Next Steps
- Explore more Big Data use cases
- Stay tuned for next tips in this series to learn more about Big Data ecosystem