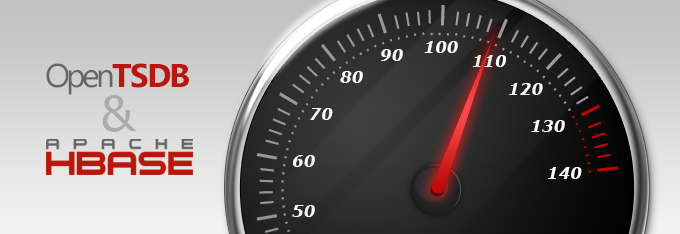
OpenTSDB and HBase rough performance test
In order to see what technological choices we have to implement a charting solution for hundreds of millions of points we decided to try OpenTSDB and check results against its underlying HBase.The point of this test is to get a rough idea if this technology would be appropriate for our needs. Planned the following tests:
- fastest data retrieval to get 5000 points out of 10 million points,
- fastest data retrieval to get 5000 points out of 200 million points.
OpenTSDB v2.0 Benchmark
From the OpenTSDB site, the description is:OpenTSDB is a distributed, scalable Time Series Database (TSDB) written on top of HBase. OpenTSDB was written to address a common need: store, index and serve metrics collected from computer systems (network gear, operating systems, applications) at a large scale, and make this data easily accessible and graphable.
Retrieval of 5000 out of 10 million points
System, configuration and data retrieval procedure
The benchmark machine is Linux (Ubuntu 12.04 64-bit) with 4 Cores and 10GB of RAM.With OpenTSDB v2.0 used HBase version 0-94.5. Disabled compression (COMPRESSION = NONE) because had problems with compression=lzo on Ubuntu, it happened from time to time to receive errors on database creation.
On OpenTSDB put the data through socket and got the data through the OpenTSDB http api.
Generated an OpenTSDB database with 10 million records by inserting into a metric: a long int date, an int value, and a tag named “sel” that is a string.
The insert operation is made with one thread. For data
retrieval used threads (1,2,4 and 8 threads per run), in our test case
every thread runs the same operation.
5000 rows selected out of 10 million rows
Database | Operation | Total rows | Threads | No of selected rows | Run time (Select + Fetch) | |
OpenTSDB | Insert | 10000000 | 1 | 0 | 127305 ms | |
OpenTSDB | Select+Fetch | 10000000 | 1 | 5000 | 2224 ms | no cache |
OpenTSDB | Select+Fetch | 10000000 | 1 | 5000 | 161 ms | |
OpenTSDB | Select+Fetch | 10000000 | 2 | 5000 | 146 ms | |
OpenTSDB | Select+Fetch | 10000000 | 4 | 5000 | 237 ms | |
OpenTSDB | Select+Fetch | 10000000 | 8 | 5000 | 228 ms |
threads – represents the number of threads that ran in the same time
first run – it’s the first run without any cache made, the other runs are with cache
run time – it’s the total run time of select+fetch
Problems encountered
While using OpenTSDB encountered the following problems:- The retrieved data at this moment can be only as ASCII (raw data) or as png image. The JSON option it’s not yet implemented,
- Failed to run the test case with 200 million points inserted into a metric, even when runing the OpenTSDB Java instance with 10GB of RAM (-Xmx10240m -Xms10240m -XX:MaxPermSize=10g ) always receieved an OutOfMemory error. Received this error from OpenTSDB logs not from HBase or our Java process,
- On OpenTSDB if you insert 2 points with the same date in the same metric (in seconds) all queries that will include the duplicate date will fail with an exception (net.opentsdb.core.IllegalDataException: Found out of order or duplicate data),
- The connection to the HBase server dropped suddenly several times,
- Not an error but maybe a limitation: when tried inserting 10 million metrics got an “[New I/O worker #1] UniqueId: Failed to lock the `MAXID_ROW’ row”.
Conclusions for this test case
OpenTSDB beats MySql and
MongoDB at every test. It is 2-4X faster than MySql with or without
cache, 7 – 328X times faster than MongoDB.
The problems encountered at the current version show that this version can’t be used in production, needs fixes.
Retrieval of 5000 out of 200 million points
As stated in the “Encountered problems” section of the previous test it was not possible to test performance for 200 million points due to OutOfMemory errors.Failed to run the test case with 200 million points, even when runing the OpenTSDB Java instance with 10GB of RAM (-Xmx10240m -Xms10240m -XX:MaxPermSize=10g ) always receieved an OutOfMemory error. Received this error from OpenTSDB logs not from HBase or our Java process
Code used for tests
Insert Code:HBase Test
Made this test mostly as a check on the previous OpenTSDB performance results.On Hbase inserted into rowKey (in this case “ubuntu” or “another”): String family, String qualifier and String Value
database | operation | total rows | threads | no of selected rows | run time (select + fetch) | |
HBase | Insert | 10000000 | 8 | 0 | 4285229 ms | |
HBase | Select+Fetch | 10000000 | 1 | 5000 | ms | no cache |
HBase | Select+Fetch | 10000000 | 1 | 5000 | 134 ms | |
HBase | Select+Fetch | 10000000 | 2 | 5000 | 184 ms | |
HBase | Select+Fetch | 10000000 | 4 | 5000 | 337 ms | |
HBase | Select+Fetch | 10000000 | 8 | 5000 | 257 ms |
OpenTSDB v1.1.0 Benchmark
Retrieval of 5000 out of 10 million points
System, configuration and data retrieval procedure
The benchmark machine is Linux (Ubuntu 12.04 64-bit) with 4 Cores and 10GB of RAM.For the LZO compression built hadoop-lzo and copied the lib to the HBase instance. Also created the tsdb tables with COMPRESSION=lzo.
On OpenTSDB put the data through socket and got the data through the OpenTSDB http api.
5000 rows selected out of 10 million rows with LZO
Database | Operation | Total rows | Threads | No of selected rows | Run time (Select + Fetch) | |
OpenTSDB 1.1.0 | Insert | 10000000 | 1 | 0 | 113651 ms | |
OpenTSDB 1.1.0 | Select+Fetch | 10000000 | 1 | 5000 | 2895 ms | no cache |
OpenTSDB 1.1.0 | Select+Fetch | 10000000 | 1 | 5000 | 97 ms | |
OpenTSDB 1.1.0 | Select+Fetch | 10000000 | 2 | 5000 | 140 ms | |
OpenTSDB 1.1.0 | Select+Fetch | 10000000 | 4 | 5000 | 128 ms | |
OpenTSDB 1.1.0 | Select+Fetch | 10000000 | 8 | 5000 | 207 ms |
threads – represents the number of threads that ran in the same time
run time – it’s the total run time of select+fetch
5000 rows selected out of 10 million rows with Date Range
Inserted 10 million points (5000 points in 2013 and the rest before 2013). Made a query for 5000 points with max value from 2013/01/03-12:00:00 – current date
Database | Operation | Total rows | Threads | No of selected rows | Run time (Select + Fetch) | |
OpenTSDB 1.1.0 | Insert | 10000000 | 1 | 0 | 124185 ms | |
OpenTSDB 1.1.0 | Select+Fetch | 10000000 | 1 | 5000 | 136 ms | no cache |
OpenTSDB 1.1.0 | Select+Fetch | 10000000 | 1 | 5000 | 126 ms | |
OpenTSDB 1.1.0 | Select+Fetch | 10000000 | 2 | 5000 | 170 ms | |
OpenTSDB 1.1.0 | Select+Fetch | 10000000 | 4 | 5000 | 179 ms | |
OpenTSDB 1.1.0 | Select+Fetch | 10000000 | 8 | 5000 | 227 ms |
Problems encountered
While using OpenTSDB 1.1.0 encountered the following problems:- When tried to insert 200 million points with the default parametrs (./tsdb tsd –port=4242 –staticroot=staticroot –cachedir=”$tsdtmp”) got an OutOfMemory exeception after a while,
- When tried to insert 200 million points by modifying the startup of
the tsdb to have have access to more RAM (java -Xmx10240m -Xms10240m
-XX:MaxPermSize=10g -enableassertions -enablesystemassertions -classpath
/root/opentsdb-1.1.0/third_party/hbase/asynchbase-1.4.1.jar:/root/opentsdb-1.1.0/third_party/guava/guava-13.0.1.jar:/root/opentsdb-1.1.0/third_party/slf4j/log4j-over-slf4j-1.7.2.jar:/root/opentsdb-1.1.0/third_party/logback/logback-classic-1.0.9.jar:/root/opentsdb-1.1.0/third_party/logback/logback-core-1.0.9.jar:/root/opentsdb-1.1.0/third_party/netty/netty-3.6.2.Final.jar:/root/opentsdb-1.1.0/third_party/slf4j/slf4j-api-1.7.2.jar:/root/opentsdb-1.1.0/third_party/suasync/suasync-1.3.1.jar:/root/opentsdb-1.1.0/third_party/zookeeper/zookeeper-3.3.6.jar:/root/opentsdb-1.1.0/tsdb-1.1.0.jar:/root/opentsdb-1.1.0/src
net.opentsdb.tools.TSDMain –port=4242 –staticroot=staticroot
–cachedir=/tmp/tsd/tsd) got often the following exception:2013-04-03
18:07:18,965 ERROR [New I/O worker #10] CompactionQueue: Failed to write
a row to re-compact
org.hbase.async.RemoteException: org.apache.hadoop.hbase.RegionTooBusyException: region is flushing
at org.apache.hadoop.hbase.regionserver.HRegion.checkResources(HRegion.java:2592)
…, - If tried to query the data from point 2. got the following
exception:java.lang.ArrayIndexOutOfBoundsException: nullopentsdb process
java.lang.OutOfMemoryError: GC overhead limit exceeded, - If in the same metric there are two identical dates got the following exception (and didn’t get any data from OpenTSDB):
Error 2
Duplicate data cell:
Request failed: Internal Server Error
net.opentsdb.core.IllegalDataException: Found out of order or duplicate data: cell=Cell([56, 87], [0, 0, 0, 0, 0, 1, 12, -112]), delta=901.
Conclusions for this test case
The 1.1.0 is more stable than 2.0, didn’t crash but gave the above exceptions.
Seems
that the LZO option doesn’t make the insert and no cache retrieval
faster for this test case. Some improvements can be seen on the data
retrieval with cache.
Got a surprisingly good value on the date range query with no cache.
Code used for tests
Insert Code:
1
2
3
4
5
6
7
8
9
10
11
12
13
14
|
URL start = new URL(
"http://localhost:4242/q?start=2013/01/03-12:00:00&m=max:test&ascii");
URLConnection yc = start.openConnection();
BufferedReader in = new BufferedReader(new InputStreamReader(
yc.getInputStream()));
String inputLine;
while ((inputLine = in.readLine()) != null) {
count++;
// System.out.println(inputLine);
}
in.close();
System.out.println("Rows: " + count);
|